p/s It’s interactive~ You can play around!
This was a dataset recording the sales records of a particular supermarket in Myanmar, over specific time periods. There were 17 columns in the dataset, and for the purposes of data visualization / Business Intelligence above throughout MS PowerBI, I had created a total of 7 new columns, which were being treated and used as new features, as well as a total of 8 new measures, mainly performing some specific calculations.
Let’s get started with some samples I analyzed!
data was there, waiting our transformation
Male Customers Patterns
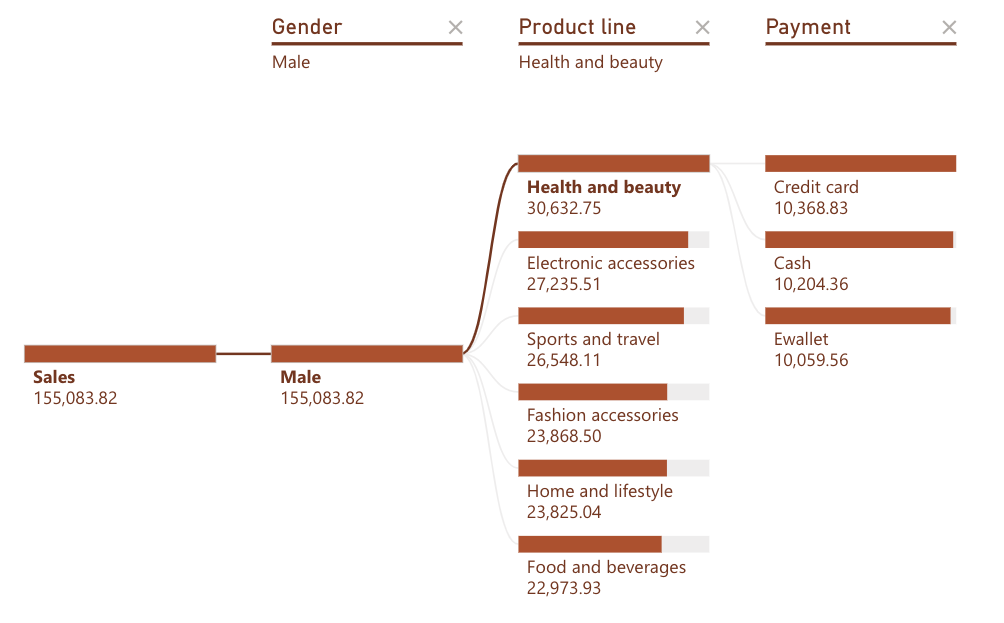
Observation
FrFrom the first glance, we can notice the bestselling products for male customers are under the category Health and Beauty. The sales achieved was as high as 30 thousand dollars in total, comparatively with the rest. Then, we further broke down the analysis by using payment method demographics and get known each of them were sharing similar values. Same method can be used to observe the rest in the product category.
Analysis
We knew the payment methods did not differ significantly, hence we suggest in future, all the Sales, Special Events, Discounts, Promotions, or equivalent events, in neither e-wallet platform nor retail shops, might not attract male customers, or affect their purchasing behaviors. Sales and Marketing teams are suggested to move on to other factors in order to boost the sales in Male Customer Category, instead of focusing on online payments platforms.
Female Customers Patterns
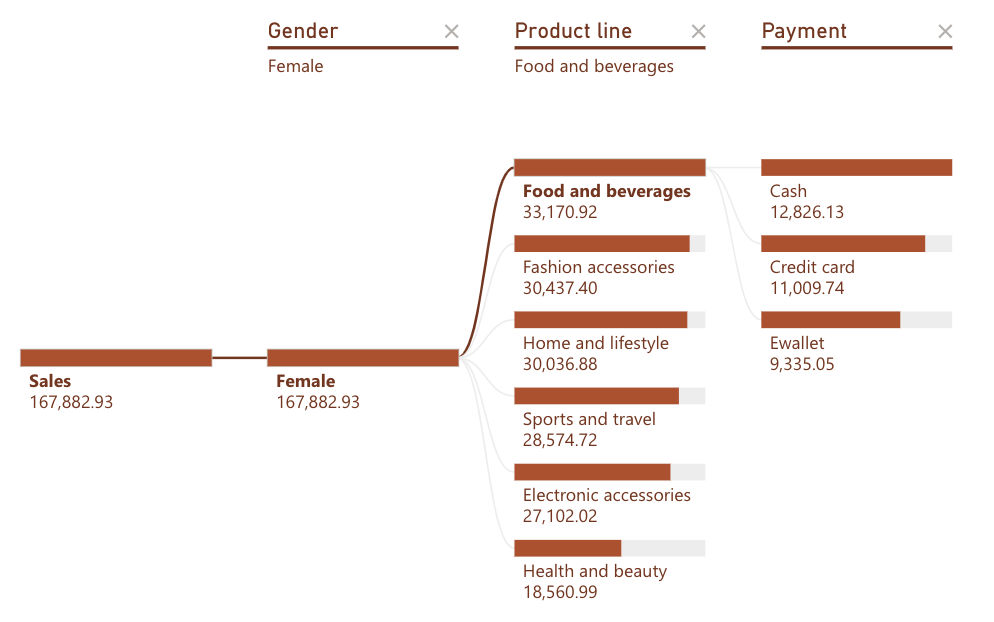
Observation
For female customers, the products with the highest sales fell under Category Food and Beverages The products with the highest sales fell under Category Food and Beverages, achieving a total of 33 thousand dollars’ worth of sales, followed by Fashion Accessories and Lifestyle categories. When we further broke down, we noticed that female customers had a tendency of using cash in purchasing goods, followed by cards and e-wallet.
Analysis
The top sales were under Food and Beverage, we could say that majority of the female customers, happen in supermarkets, might be the one in-charge in purchasing daily needs, say, food. Housewives might be the majority individual in purchasing so, such that goods like raw meats, seafoods, vegetables, fruits, basically consumable groceries, are their priority towards their family’s necessities.
Next, there was a great amount of records stating female customers did spend a lot by using cash for food. We all knew food is a daily need, and the respective price must be affordable to anyone, hence female customers could have bought very few, or just enough food in each of their purchases, resulting in a not-so-high total price when checkout. So, the transaction with such a low price to be paid mostly ends up with cash tendered for the quicker process, and lesser procedures.
Peak Timing
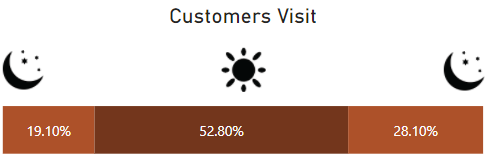
Observation
From solely a column describing the transaction time in the supermarkets, we came up with such a visualization that showed which time had most of the customers visited. The peak moments meant to happen during daytime, which was between 12pm to 6pm.
Analysis
It was understandable that It was understandable that the majority of customers visited the shops during daytime, even the number of visits in evenings, recorded between 6pm to 12am, was more than the number in the morning time. It is suggested that the shop retailers could assign sufficient work forces and more focus in each section in the respective time, while too all the promotions and sales are recommended to be carried out during the peak time to reach a better retention.
Too, please feel free to choose different filters in the interactive dashboard in the very top there of the webpage, to see the results fell under different categories! Some head-ups for you, Branch C had the most number of visitors throughout the night!
Sale
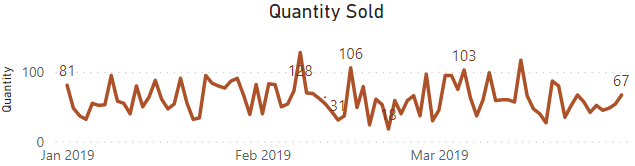
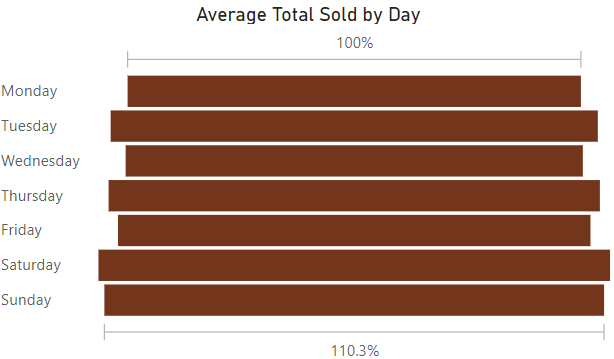
Observation
From Figure 4, we noticed there were some peak moments, which happened on 8th of January, 7th and 15th of February as well as 14th of March. These dates happened in the early, or middle of the months.
In the average goods sold from Figure 5, we could see the weekend, Saturday and Sunday, had the 2 highest records of total value of the goods sold, comparatively with other days.
Analysis
When we chased back the exact date from the calendar, we realized 7th February, the highest number of total sales on record, was the third day of Chinese New Year’s Festival. In Asian countries, Chinese New Year is treated as one of the major festivals and most of the time, the first and the second day is defaulted as a public holiday. Hence, the third day of CNY had recorded the highest sales records, we noticed the shops might have special festival discounts or promotion sales that time.
For the rest of the dates, 8th January was Monday, 15th February and 14th March was Thursday and Friday respectively. There were no coincidences nor festivals spotted in these dates, moreover they fell under ordinary weekdays. We could make a hypothesis such that shop sellers could trace back if those dates having new arrivals, or stock clearance happened.
For Figure 5, we saw that the highest total sales happened on weekends. Hence, we suggested that for all advertisements, new arrivals or events which intend for attention, shop sellers could hold them on weekends. For a rather clearer indicator, one can choose a branch specific by simply filtering it, to see the respective result.
Conclusion
This dataset had demonstrated quite consistent results in overall perspective, that most of the time, customers behaviors were most likely similar. All recommendations and suggestions above are meant to improve and maximize, particularly the sales, exposure, reputations, services, availability etc. of the shops, or say, the company. I wish those words are really helping and making sense in perspective of Sales and Marketing!
Finally the sample showcases and analysis for this particular report and topic might’ve stopped here, not limit to further going to another topics and dataset, of course! Please dear, feel free, don’t hesitate to leave a comment or insight below!
C’ya!